Instructors
Two half-day courses will be delivered in series separated by a lunch break.
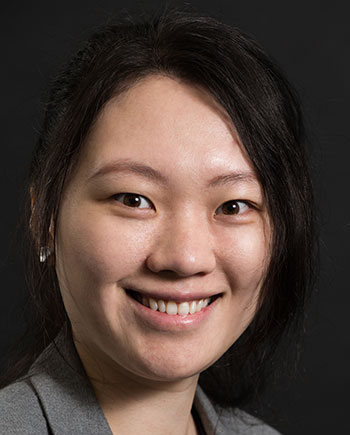
“Machine Learning-based Process Modeling and Monitoring”
Dr. Ying Lin, Assistant Professor, Industrial Engineering, University of Houston
Timely monitoring of a complex and dynamic process is critical for abnormal event detection, prevention, and control. The rapid advances in sensing and information technology have provided unprecedented information infrastructure, holding great promises to enable efficient process modeling and monitoring technologies being developed in a variety of applications, including healthcare and advanced manufacturing. However, there exist numerous longstanding challenges in process modeling and monitoring including 1) the complex dynamics in process progression and the lack of real-time observed high-quality measurements; 2) the sparse and inadequate monitoring data within a single process; 3) the difficulties in process modeling and monitoring under limited monitoring or sensing resources. To mitigate these challenges, this short course will introduce state-of-the-art machine learning techniques for 1) integrative process modeling and monitoring based on multivariate noisy sensing data fusion; 2) collaborative process modeling and monitoring for a population of dependent processes; 3) adaptive process modeling and monitoring that proactively allocates the limited monitoring resources to the most severe or high-risk processes. This short course will also introduce the applications of these techniques to the advanced superconductor manufacturing process and chronic disease modeling and monitoring.
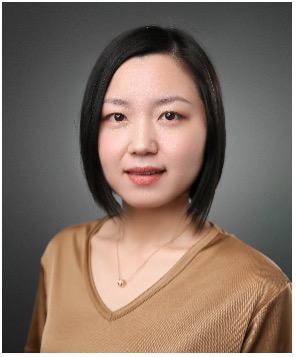
“Fair Machine Learning in Healthcare”
Dr. Na Zou, Corrie & Jim Furber 64' Faculty Fellow, Department of Engineering Technology and Industrial Distribution, Texas A&M University
Due to the digitization of healthcare data and advancements in computing power, machine learning methods are increasingly being used in the healthcare domain. When used in healthcare applications, machine learning methods inherit or even amplify existing biases in data, leading to fairness issues such as unequal distribution of healthcare resources or disparity in diagnostic accuracy across demographic groups. Therefore, addressing the issue of fairness has recently attracted increasing attention from the healthcare machine learning community. In this short course, we will bridge the gap between fair machine learning and healthcare disparities by defining the problem of fairness in healthcare, identifying its sources, and categorizing fair machine learning algorithms. Specifically, we define two types of fairness problems in healthcare based on distributive justice and categorize the corresponding measurements from a machine learning perspective. Then, we will introduce the various biases that exist, as well as the corresponding machine learning mitigation algorithms, along the stages in the machine learning life cycle. We will conclude by identifying additional challenges to the current fairness issue with machine learning in healthcare and outlining several promising new directions.